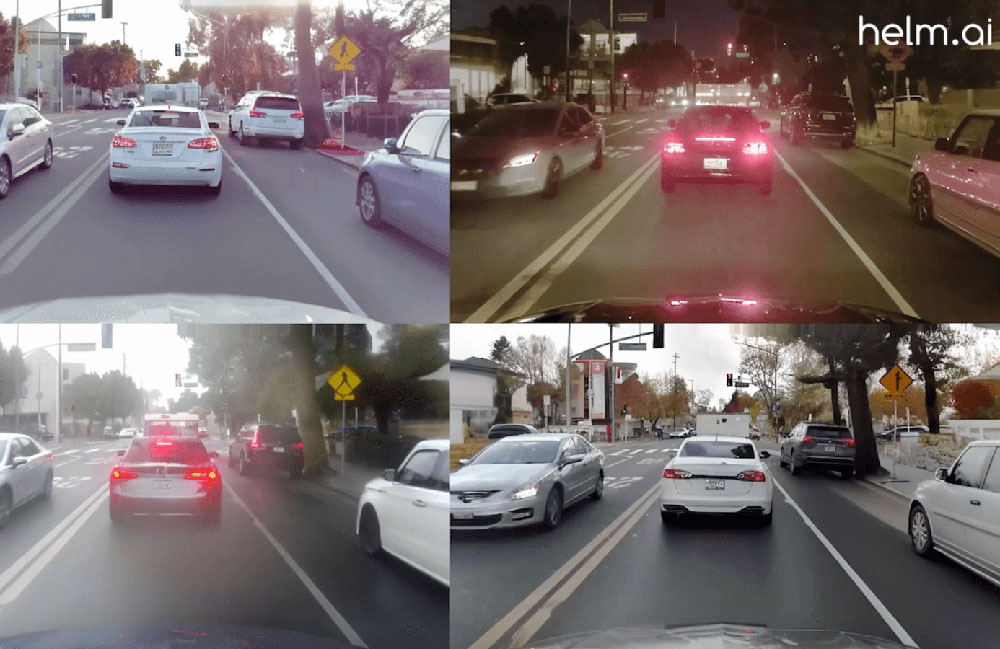
Helm.ai’s GenSim-2 permits customers to change video information utilizing generative AI. | Supply: Helm.ai
Autonomous car builders may quickly use generative AI to get extra out of the info they collect on the roads. Helm.ai this week unveiled GenSim-2, its new generative AI mannequin for creating and modifying video information for autonomous driving.
The corporate stated the mannequin introduces AI-based video modifying capabilities, together with dynamic climate and illumination changes, object look modifications, and constant multi-camera help. Helm.ai stated these developments present automakers with a scalable, cost-effective system to counterpoint datasets and deal with the lengthy tail of nook circumstances in autonomous driving improvement.
Skilled utilizing Helm.ai’s proprietary Deep Educating methodology and deep neural networks, GenSim-2 expands on the capabilities of its predecessor, GenSim-1. Helm.ai stated the brand new mannequin permits automakers to generate various, extremely real looking video information tailor-made to particular necessities, facilitating the event of sturdy autonomous driving programs.
Based in 2016 and headquartered in Redwood Metropolis, CA, the firm develops AI software program for ADAS, autonomous driving, and robotics. Helm.ai presents full-stack real-time AI programs, together with deep neural networks for freeway and concrete driving, end-to-end autonomous programs, and improvement and validation instruments powered by Deep Educating and generative AI. The corporate collaborates with international automakers on production-bound initiatives.
Helm.ai has a number of generative AI-based merchandise
With GenSim-2, improvement groups can modify climate and lighting circumstances reminiscent of rain, fog, snow, glare, and time of day (day, night time) in video information. Helm.ai stated the mannequin helps each augmented actuality modifications of real-world video footage and the creation of absolutely AI-generated video scenes.
Moreover, it permits customization and changes of object appearances, reminiscent of highway surfaces (e.g., paved, cracked, or moist) to autos (sort and coloration), pedestrians, buildings, vegetation, and different highway objects reminiscent of guardrails. These transformations will be utilized persistently throughout multi-camera views to boost realism and self-consistency all through the dataset.
“The power to control video information at this stage of management and realism marks a leap ahead in generative AI-based simulation expertise,” stated Vladislav Voroninski, Helm.ai’s CEO and founder. “GenSim-2 equips automakers with unparalleled instruments for producing excessive constancy labeled information for coaching and validation, bridging the hole between simulation and real-world circumstances to speed up improvement timelines and cut back prices.”
Helm.ai stated GenSim-2 addresses business challenges by providing a substitute for resource-intensive conventional information assortment strategies. Its skill to generate and modify scenario-specific video information helps a variety of functions in autonomous driving, from creating and validating software program throughout various geographies to resolving uncommon and difficult nook circumstances.
In October, the corporate launched VidGen-2, one other autonomous driving improvement device based mostly on generative AI. VidGen-2 generates predictive video sequences with real looking appearances and dynamic scene modeling. The up to date system presents double the decision of its predecessor, VidGen-1, improved realism at 30 frames per second, and multi-camera help with twice the decision per digicam.
Helm.ai additionally presents WorldGen-1, a generative AI basis mannequin that it stated can simulate the complete autonomous car stack. The corporate stated it could generate, extrapolate, and predict real looking driving environments and behaviors. It may generate driving scenes throughout a number of sensor modalities and views.